Digital Twins
Within the business community (Marr, 2017) the metaphor of a “Digital Twin” is gaining popularity as a way to explain the potential of IoT-based assets and smart environments. A digital twin refers to a digital replica of physical assets (car), processes (value-chain), system, or physical environment (building). The digital representation provided by the digital twin can be analysed to optimise the operation of the “physical twin”. The digital twin provides a digital representation (i.e. simulation model, data-driven model) that updates and changes as the physical twin changes. Digital twins can provide digital representations ranging from human organs such as the heart and lungs to aircraft engines and city-scale twins. For example, the SmartSantander smart city project has deployed tens of thousands of Internet-connected sensor devices in large cities across Europe (Cheng et al., 2015). The sensing capabilities of these devices are wide-ranging, including solar radiation, wind speed and direction, temperature, water flow, noise, traffic, public transport, rainfall, parking, and others. The devices provide a digital representation of the state of the real-world, in the case of SmartSantander a digital representation of the city, which enables visibility into processes and operations of the city that can be analysed and optimised.

With the use of advanced analytics and artificial intelligence techniques, the digital twin can learn the optimal operating conditions of the physical twin and optimise the physical twins’ operations in areas such as performance, maintenance, and user experience. One of the most promising outputs from such an analysis is the possibility to find root-causes of potential anomalies which can happen (prediction) and improve the physical process (innovation).
Digital twins are a sophisticated example of a cyber-physical system which is constructed from multiple sources of data including real-time IoT sensors, historical sensor data, traditional information systems, and human-in-the-loop input from human operators and domain and industrial experts. The core of a digital twin requires a holistic and systematic approach to data management and decision-making, at the heart of a digital twin is an OODA Loop.
Enabling OODA for Digital Twins and Intelligent Applications
We use the OODA Loop to align the different development phases of digital twins and intelligent applications with the relevant RLD support services.

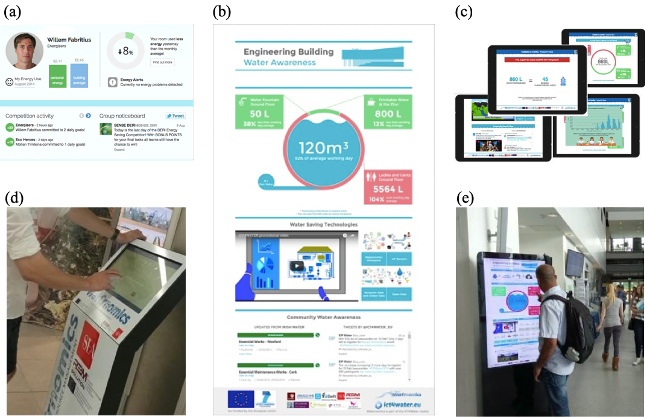

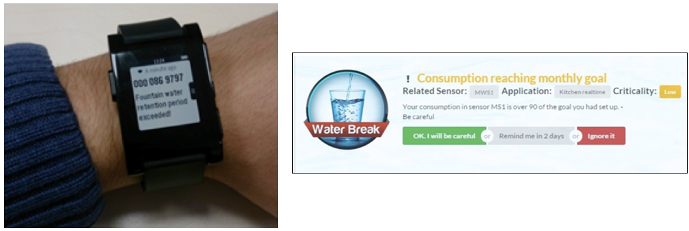
An IoT-enhanced Journey for Smart Energy and Water
There is a significant opportunity to improve energy and water resource management and conservation by analysing, designing, and implementing intelligent systems to increase demand and supply efficiency. To manage energy and water holistically within a smart environment, it is essential to use decision support tools that present meaningful and contextual information about usage, price, and availability of energy and water intuitively and interactively to users. Smart environments by leveraging IoT can support the development of intelligent applications for efficient and effective management of the resource within the environment. Users will need different information to manage their energy and water consumption, from home users managing their personal water usage, business users managing the water consumption of their commercial activities, to municipalities managing regional distribution and consumption at the level of a city or a region. To deliver a completing user experience for these diverse users, it is necessary to leverage knowledge from several different sources, from IoT-based devices to contextual data sources (including environmental impacts, water quality, energy usage, end-user feedback, occupancy patterns and meteorological data). This mass of data needs to be analysed to extract insights which then must be packaged within a set of personalised applications, designed within the context of a holistic user journey for the targeted user.
Over the past years, we have been involved in a number of projects (Curry et al., 2013) (Aggarwal, Ashish and Sheth, 2014) (Curry et al., 2014) concerned with investigating the use of next-generation information platforms for smart environments, specifically targeting intelligent energy and water management systems. The five pilot smart environments are detailed in Chapter 14. Within these projects, we have developed user application following the model IoT-enhanced user experiences detailed in the previous section. Within our pilots, the model was implemented using a Real-time Linked Dataspace for the digital-side and behaviour change theories for the HCI-side.
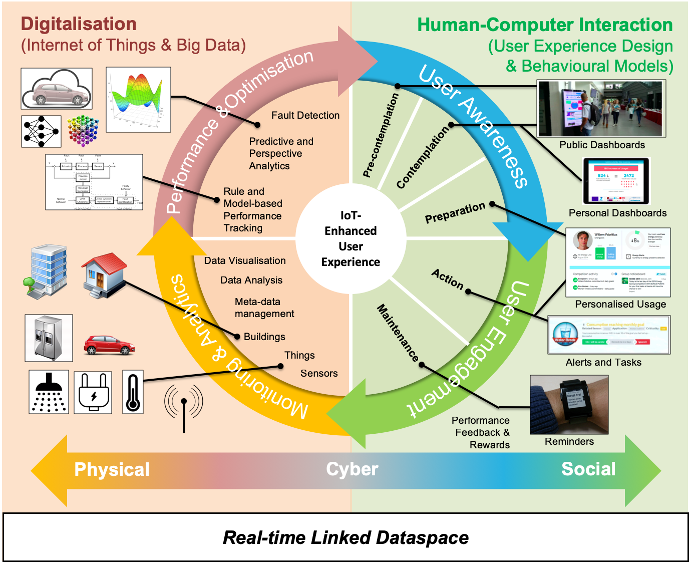
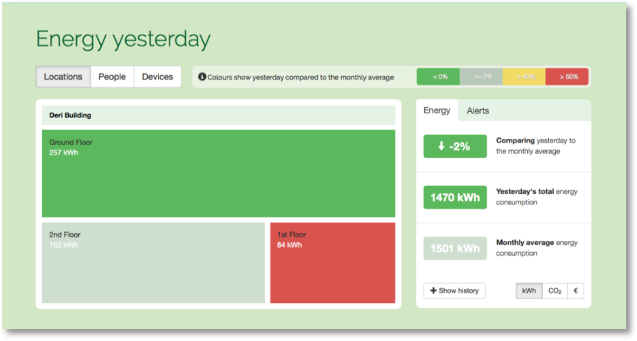
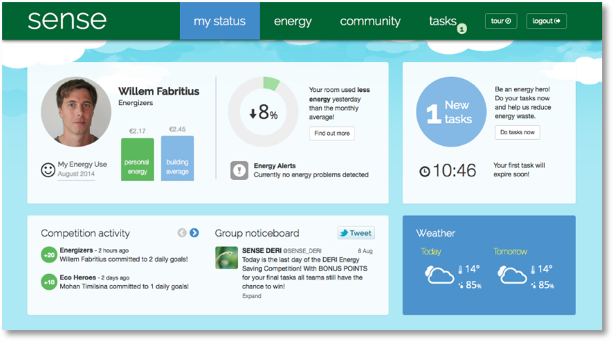
Further details are available from the Waternoics.eu project website.
Excerpts from:
- Curry E. (2020) Enabling Intelligent Systems, Applications, and Analytics for Smart Environments Using Real-time Linked Dataspaces. In: Real-time Linked Dataspaces. Springer, Cham
- Curry E., Derguech W., Hasan S., Kouroupetroglou C., ul Hassan U., Fabritius W. (2020) Building Internet of Things-Enabled Digital Twins and Intelligent Applications Using a Real-time Linked Dataspace. In: Real-time Linked Dataspaces. Springer, Cham
- Curry E., Fabritius W., Hasan S., Kouroupetroglou C., ul Hassan U., Derguech W. (2020) A Model for Internet of Things Enhanced User Experience in Smart Environments. In: Real-time Linked Dataspaces. Springer, Cham
References
Aggarwal, C. C., Ashish, N. and Sheth, A. (2014) ‘The internet of things: A survey from the data-centric perspective’, in Managing and Mining Sensor Data, pp. 383–428. doi: 10.1007/978-1-4614-6309-2_12.
Boyd, J. (1987) ‘A discourse on winning and losing’, Air University document MU43947, briefing, vol. 1,. Available at: http://www.airuniversity.af.mil/Portals/10/AUPress/Books/B_0151_Boyd_Discourse_Winning_Losing.PDF (Accessed: 28 June 2018).
Cheng, B. et al. (2015) ‘Building a Big Data Platform for Smart Cities: Experience and Lessons from Santander’, in Proceedings – 2015 IEEE International Congress on Big Data, BigData Congress 2015. doi: 10.1109/BigDataCongress.2015.91.
Curry, E. et al. (2013) ‘Linking building data in the cloud: Integrating cross-domain building data using linked data’, Advanced Engineering Informatics, 27(2), pp. 206–219. Available at: http://www.edwardcurry.org/publications/Curry_AEI_2013.pdf.
Curry, E. et al. (2014) ‘Linked Water Data For Water Information Management’, in 11th International Conference on Hydroinformatics (HIC 2014). New York, New York, USA. Available at: http://www.edwardcurry.org/publications/Curry_HIC2014.pdf.
Curry, E. et al. (2018) ‘Internet of Things Enhanced User Experience for Smart Water and Energy Management’, IEEE Internet Computing, 22(1), pp. 18–28. doi: 10.1109/MIC.2018.011581514.
Curry, E. et al. (2019) ‘A Real-time Linked Dataspace for the Internet of Things: Enabling “Pay-As-You-Go” Data Management in Smart Environments’, Future Generation Computer Systems, 90, pp. 405–422. doi: 10.1016/j.future.2018.07.019.
Marr, B. (2017) ‘What Is Digital Twin Technology – And Why Is It So Important?’, Forbes.